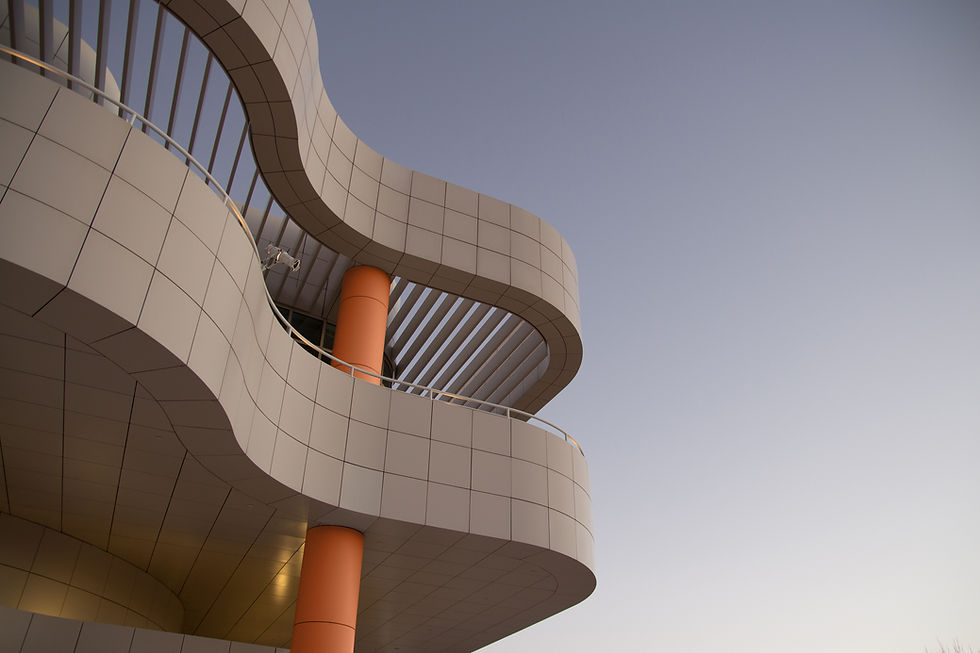
Turn Nasty Data into Gold: Expert Remediation for Usable Assets
Jul 19, 2024
5 min read
0
12
0
What is bad data?

Bad data refers to data that is inaccurate, incomplete, inconsistent, or unreliable, and it can significantly undermine the effectiveness of data-driven decision-making and analysis. Here’s a breakdown of what constitutes bad data and the problems it can cause:
Types of Bad Data
Inaccurate Data
Errors: Data that contains incorrect values due to human errors, system glitches, or faulty data entry. For example, a typo in a customer's contact information.
Misleading Information: Data that is not representative of reality or context. For instance, outdated market research data that no longer reflects current trends.
Incomplete Data
Missing Values: Data with gaps or missing entries. For example, a database of customer profiles where some records lack essential information such as email addresses.
Unfinished Records: Data that is partially recorded or incomplete, which can lead to incorrect analyses or conclusions.
Inconsistent Data
Conflicting Information: Data that has contradictions or discrepancies. For example, a customer's address recorded differently in two separate systems.
Format Issues: Data that is not standardised, such as dates recorded in different formats (MM/DD/YYYY vs. DD/MM/YYYY).
Outdated Data
Stale Data: Information that has not been updated to reflect recent changes. For example, a sales database that hasn’t been refreshed with the latest transactions.
Duplicate Data
Redundant Entries: Multiple records representing the same entity. For example, having several entries for the same customer due to multiple sign-ups.
Irrelevant Data
Non-essential Information: Data that does not contribute to the analysis or decision-making process. For instance, collecting data that is unrelated to the business objectives.
What caused bad data?

Bad data is often the result of several underlying causes that compromise its accuracy and reliability. Human error is a primary factor, where mistakes during data entry or processing lead to incorrect or incomplete information. System glitches or technical malfunctions can corrupt data during storage or transmission, introducing inaccuracies. Lack of standardisation in data collection and input processes can result in inconsistent formats and interpretations, leading to discrepancies. Inadequate data validation mechanisms may fail to catch errors or invalid entries, allowing flawed data to persist. Poor integration of data from multiple sources can create inconsistencies and duplicates, especially when systems do not align in terms of data formats or definitions. Additionally, outdated data can arise from the failure to regularly update or cleanse datasets, causing the information to become irrelevant over time. Addressing these root causes requires implementing robust data governance practices, improving system reliability, and ensuring rigorous data validation and standardisation protocols.
What is the cost of bad data?
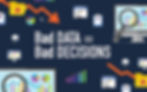
The cost of poor data quality can be substantial, affecting organisations across multiple dimensions. Operationally, it leads to inefficiencies, such as increased manual efforts to correct errors and delays in processes due to inaccurate information. Financially, organisations may suffer from lost revenue due to failed transactions and billing errors, along with the direct costs of remediation efforts. Customer satisfaction can also be severely impacted, as incorrect data results in poor service experiences and eroded trust. In terms of strategic decision-making, flawed data can lead to misguided decisions and missed opportunities, undermining business objectives. Additionally, poor data quality poses risks to data-driven initiatives and marketing campaigns, often resulting in wasted resources and reduced ROI. Compliance and legal risks are heightened, with potential penalties for regulatory non-compliance and audit failures. Addressing these challenges requires a commitment to robust data quality management practices to mitigate these costs and enhance overall business performance.
How do you prevent bad data?
Preventing bad data involves implementing a combination of best practices and systematic processes to ensure data accuracy and quality. Establishing robust data governance frameworks is crucial, defining clear standards, policies, and procedures for data management. Implementing strong data validation rules and checks during data entry helps catch errors and ensure that only accurate and relevant information is captured. Standardising data formats and definitions across systems prevents inconsistencies and promotes uniformity. Regular data cleaning and maintenance are essential to identify and correct inaccuracies, remove duplicates, and update outdated information. Training employees on proper data handling and entry practices reduces human error and enhances overall data quality. Additionally, using reliable and secure systems for data storage and integration ensures that data remains accurate and protected from corruption or loss. By adopting these preventive measures, organisations can significantly reduce the risk of bad data and maintain high-quality, reliable datasets.
How about existing data issue?
In today’s data-driven world, businesses have access to an unprecedented amount of information. While we’ve made strides in preventing data issues through robust governance and validation processes, what happens when problems arise in the data we already have? Addressing existing data issues is crucial for maintaining data integrity and making informed decisions. This is where data remediation comes into play—a systematic approach to correcting and improving data quality.
What is data remediation?

Data remediation is the process of identifying, correcting, and improving data quality issues in existing datasets. It involves several key steps:
Assessment and Identification: Begin by profiling your data to identify inaccuracies, inconsistencies, and gaps. Tools and techniques such as data audits and quality assessments can help pinpoint the specific issues affecting your data.
Define Remediation Goals: Set clear objectives for what you want to achieve with the remediation process. This might include improving accuracy, completeness, or consistency across datasets.
Develop a Remediation Plan: Create a detailed plan outlining the steps required to address identified issues. Prioritise the issues based on their impact and severity, and allocate resources accordingly.
Implement Data Cleaning and Correction: Correct data errors, remove duplicates, address missing values, and standardise data formats to ensure consistency and accuracy.
Validate and Verify: After making corrections, validate the data to ensure it meets quality standards. Verification against trusted sources or cross-referencing with other datasets helps confirm accuracy.
Document and Track: Maintain detailed documentation of the remediation process, including changes made and their rationale. Tracking progress helps in assessing the effectiveness of remediation efforts.
Ongoing Management: Establish processes for continuous data quality management to prevent future issues. Regular audits and updates are essential for maintaining data integrity.
How Matrix Grid Can Help
At Matrix Grid, we understand the complexities of data remediation and the challenges associated with existing data issues. Our tailored remediation strategy is designed to address these challenges effectively. Here’s how our solution can assist you:
Customised Approach: We offer a tailored remediation strategy that aligns with your specific data environment and business needs. Our approach is designed to address the unique issues in your datasets, ensuring effective and relevant solutions.
Comprehensive Tools: Our Matrix Grid platform provides powerful tools for data profiling, cleaning, and validation. These tools help you identify and correct issues efficiently, ensuring your data is accurate and reliable.
Expert Guidance: Our team of experts provides guidance and support throughout the remediation process. From initial assessment to implementation and ongoing management, we ensure that you have the expertise needed to achieve your data quality goals.
Continuous Improvement: We focus on not just fixing current issues but also establishing processes for continuous data quality management. Our solutions help you maintain high-quality data and adapt to changing needs over time.
While preventing data issues is critical, addressing existing data problems through effective remediation is equally important. By employing a structured data remediation strategy, you can correct inaccuracies, improve data quality, and enhance your decision-making capabilities. Matrix Grid is here to support you with a tailored remediation approach that meets your specific needs and helps you achieve lasting data quality improvements.
Contact us today to learn how Matrix Grid can assist you in resolving existing data issues and ensuring that your data remains a valuable asset for your business.